A/B TESTS WITH BOOMERANG IDEAS
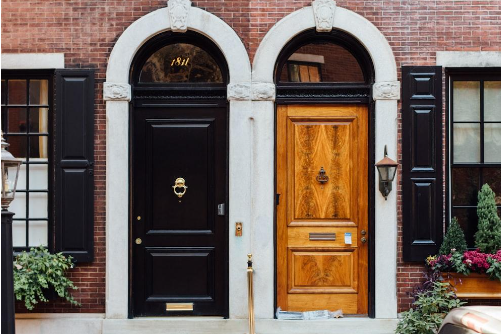
Does the color blue fit the Boomerang logo better than the color green?
Is the slogan “just do it” more convincing than the slogan “impossible is nothing”?
Are consumers more interested in a pay-per-use plan than a pay-per-month plan?
Does this ad trigger more curiosity when it is not displaying the product?
When it comes to questions like these, marketers and innovators usually decide intuitively – lacking the data to evaluate ideas more objectively. Boomerang Ideas offers a simple solution to this problem: The Boomerang A/B test allows you to measure consumer reactions to two different versions of a stimulus, observe how a proposed change (B) performs relative to a default (A), to ultimately identify the better performing option and drive change and innovation on a more objective basis.
In the following, we will…
- introduce the concept of an A/B test,
- discuss the best practices for running an A/B test,
- show how to conduct an A/B test with Boomerang Ideas,
- explain the difference between the Boomerang and the Meta A/B test, and
- present a case study to validate the Boomerang A/B test.
What is an A/B test?
An A/B test allows innovators, marketers, and researchers to objectively test their ideas for new business models, products, or marketing campaigns. It compares the performance of a control version A (the default) against a treatment version B (the proposed change). To identify the more effective solution, consumers are randomly exposed to one of these two stimuli and their reactions are measured.
The value of an A/B test lies in the gap between its expected outcome and its actual result. When relying on intuitive decision making, big investments often fail to follow through while small changes may lead to unexpectedly big profits. A/B tests thus enable a more scientific, data-based decision making and deliver numeric data to trade-off different opportunities.
A/B tests emerged for the first time in the late 1990s. By now, tech companies like Amazon, Facebook, Google, and LinkedIn conduct thousands of these experiments every year. However, smaller companies often lack the means to implement the infrastructure to collect and analyze such experimental data. Boomerang Ideas now lowers these barriers to market entry and offers an easy and affordable self-service tool to conduct simple A/B tests on social media.
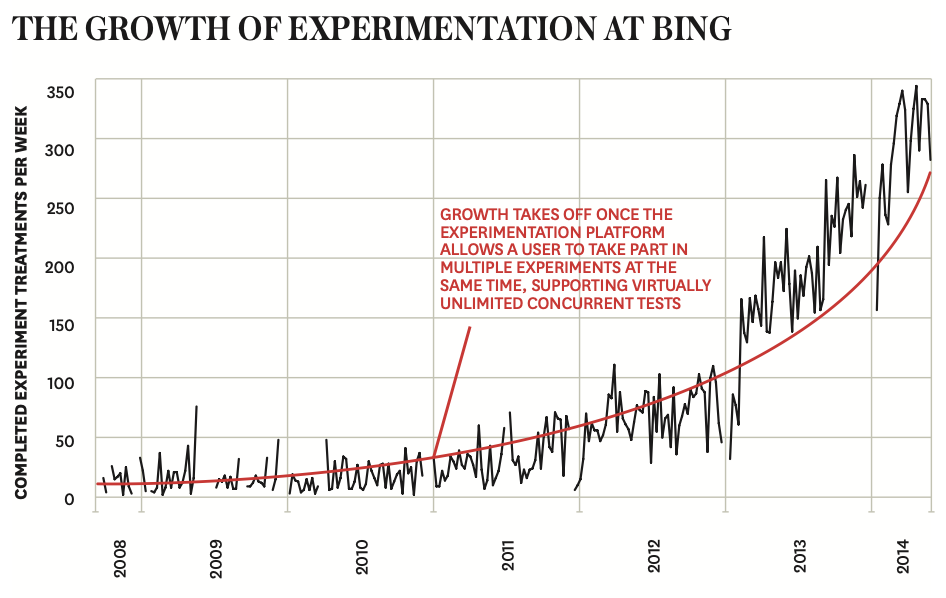
Figure 1: Big tech companies, like Bing, are running multiple experiments each week to evaluate the potential of new features, changes to their user interface, changes to their back-end, or different business models.
What are the best practices for running an A/B test?
- Identify the proposed change that you want to evaluate. To maximize the value of your test, keep the differences stimuli to a minimum. Testing too many changes at once, will make it difficult to learn about causality and conclude what specific change triggered an outcome.
- Define your target customer and figure out who you are most interested in. With Boomerang, you can restrict your audience by geographic location, age, and gender. You can also conduct your test in multiple languages. Be aware that you cannot generalize your findings from one specific group of people to the overall population.
- Specify the outcome that you are interested in and define your “overall evaluation criterion” such as consumer interest, intention to purchase, or willingness to pay. Choose a short-term measure that is most predictive for your long-term outcomes. Consider measuring further metrics to ensure that your proposed change has no unintended consequences on other outcomes.
- To avoid chance findings, try to understand why your proposed change leads to a change in outcome. Further measures might help you to uncover the causal mechanism.
How can I run an A/B test with Boomerang?
To conduct a Boomerang A/B test, go on the Boomerang website, click on ‘Throw A Boomerang’, choose a ‘Deep Dive’ Boomerang, pick the target population that you are interested in, and upgrade your journey by activating the button ‘A/B test’.
On the following page, Boomerang allows you to switch between the A and B version of your survey and adapt the question text, the visual, as well as the response options.
Once you finished designing your survey, click on the ‘Next’ button for a final check of your Boomerang specs. Now your Boomerang is ready to go!
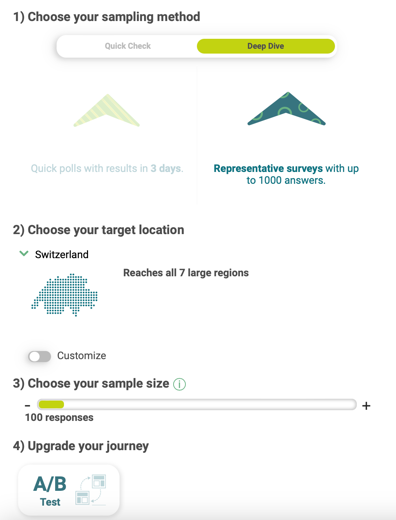

Figure 2: Run an A/B test on Boomerang, by clicking on the ‘A/B test’ button. Boomerang then allows you to switch between the A and B version of your survey to adapt the question text, the visual, as well as the response options.
What is the difference between the Boomerang and the Meta A/B test?
With the rise of A/B testing, social media platforms like Meta implemented their own A/B test tools in their ad managers. The validity of those A/B tests however has frequently been questioned.
For instance, in a Meta A/B test, the social media algorithm optimizes the display of two ads (A and B) independently. For instance, if ad A is more attractive to women and ad B is more attractive to men, the Meta algorithm will adapt the display of the two ads accordingly – showing ad A rather to women and ad B rather to men. If ad A now results in a higher number of click-troughs and likes, this outcome might be caused either by the proposed change or by the fact that women were more active on social media during the testing period.
To infer causality from an A/B test, the test needs to ensure that people are randomly assigned to either one of the two stimuli so that the two groups of people are – on average – the same. In a Boomerang A/B test, all participants hence see the same ad on their social media news feed. Only after clicking on the ad, they are randomly assigned to the A or B version of the survey. This way, the proposed change does not affect clicking behavior on social media that would in turn trigger a biased display of ads. For statistical reasons, we further recommend a sample size of at least 50 observations per survey.
A Case Study: Replicating the Anchoring Effect
To validate the Boomerang A/B test, we wanted to replicate the anchoring effect – a well-established cognitive bias proposed by Tversky & Kahneman (1974). The anchoring effect predicts that consumers are biased by a reference point (i.e., an anchor) when processing new information. For this purpose, we recruited 221 Swiss survey participants on Facebook, Instagram, LinkedIn, and Snapchat who were interested in a monthly subscription for the Swiss regional public transport.
On the second survey page, we told participants about the current price for a general monthly subscription for the Swiss public transport. Participants who received the A version of the survey read that the current price for a subscription is 349 CHF / month, i.e., the regular price for a general subscription (high anchor condition). Participants who received the B version of the survey read that the current price is 75 CHF / month, i.e., the price for an additional family member to receive a subscription (low anchor condition).
We then asked participants whether they would be willing to buy a subscription for the Swiss regional public transport for 50 CHF / month. Participants responded with “No, definitely not” (1), “No, rather not” (2), “Yes, maybe” (3), or “Yes, definitely” (4).
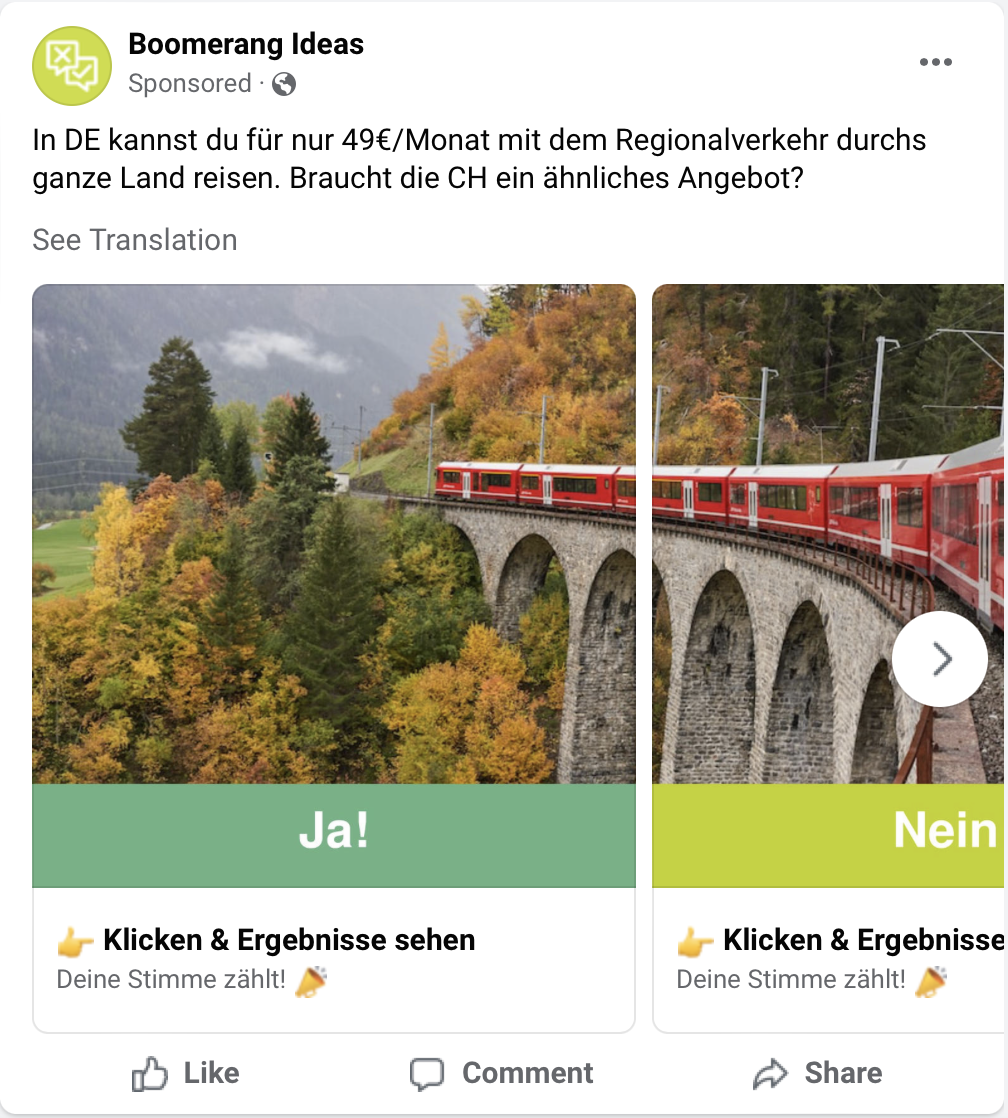
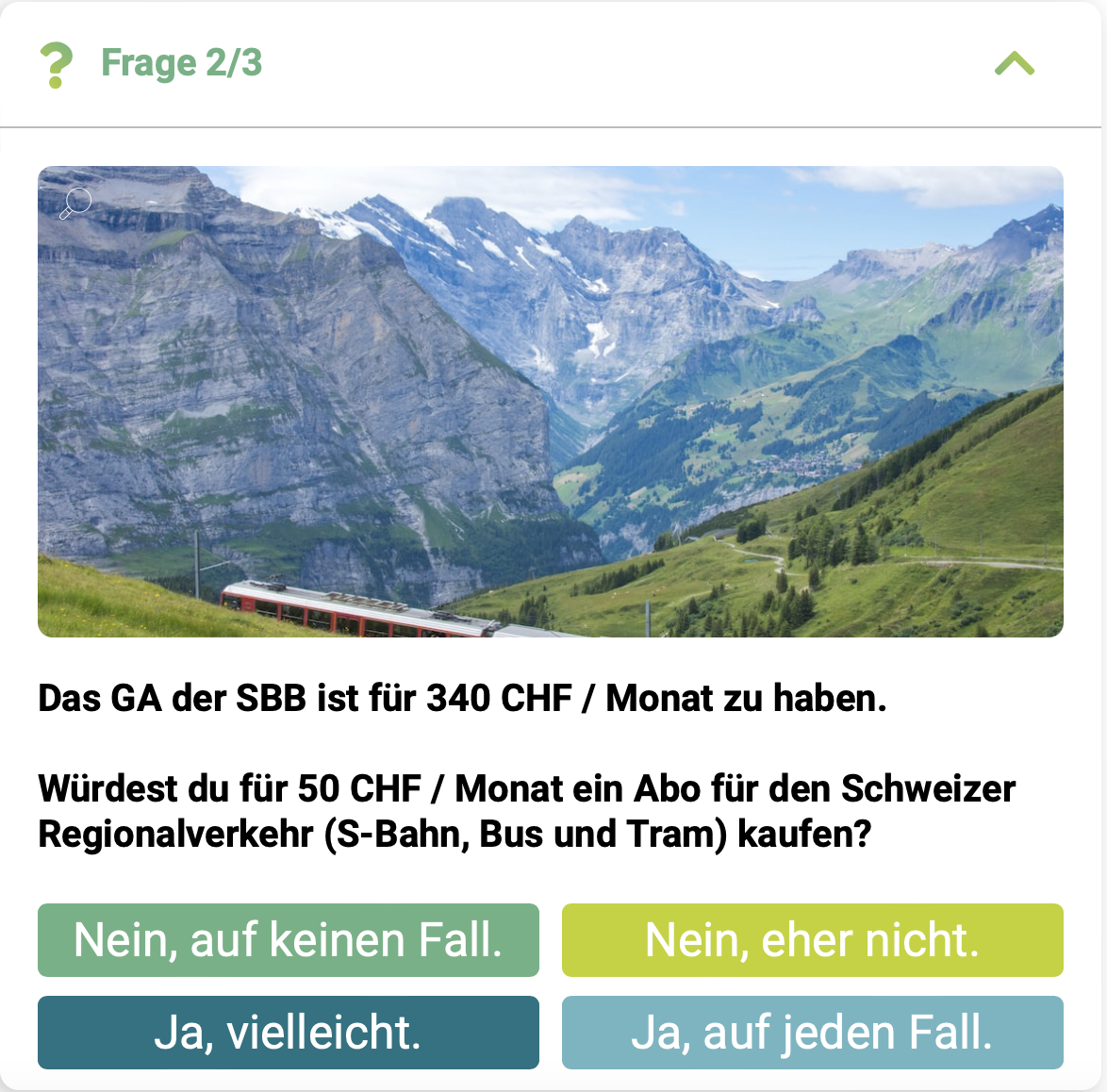
Figure 3: To validate the Boomerang A/B test tool, we replicated the anchoring effect on social media. The left side shows the Facebook ad with the first question used to recruit participants for the survey. The right side shows the second question in the A version of the survey, i.e., the high anchor condition.
A Case Study: The Results
According to the anchoring effect, we expected participants to be more interested in purchasing the 50 CHF / month subscription, when they thought that they usually pay 349 CHF / month (rather than 75 CHF / month). Even though the price offer is the same in both conditions, potential savings are perceived to be bigger in the high anchor condition. The results of our Boomerang A/B test indeed aligned with this prediction.
While most participants in the low anchor condition were “maybe interested” in the offering, most participants in the high anchor condition were “definitely interested”. Assigning numeric values from 1 (No, never) to 4 (Yes, definitely) to these responses and averaging values across survey A and survey B, we can see that participants in the low anchor condition were indeed less interested in the offering (M = 2.86) compared to users in the high anchor condition (M = 3.33). A t-test supports that this difference in means is statistically significant (p < .001).
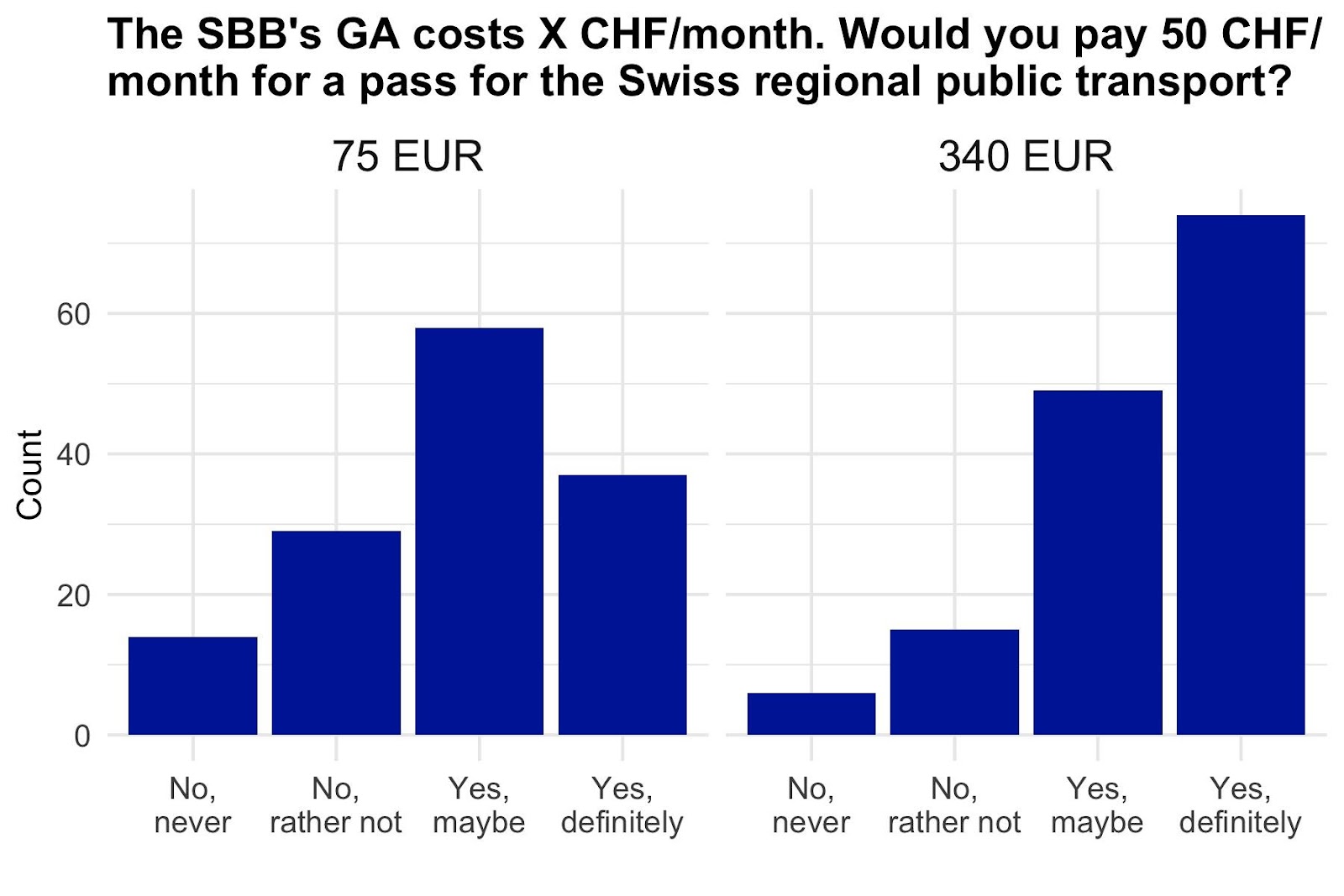
Figure 3: In line with the prediction of the anchoring effect, the Boomerang A/B test shows that consumers were more interested in the offering in the high anchor condition (340 EUR / month) compared to the low anchor condition (75 EUR / month).
A Case Study: The Randomization Check
The data further shows that the Boomerang A/B test successfully assigned participants to conditions in a random manner. There were no significant differences between conditions regarding participants’ age (p = .968), gender (p = .558), language (p = .212), or social media platform (p = .416).
We can thus conclude that the difference in consumer interest was indeed caused by the experimental manipulation (i.e., the different anchoring prices) rather than individual differences among participants (e.g., differences in age that could explain differences in public transportation preferences).
Figure 4: Participants were randomly assigned to one of the two treatment s so that there were no significant differences in age, gender, language, or social media platforms between the two groups.
Conclusion
To summarize, the Boomerang A/B test allows you to collect quantitative data to evaluate your ideas and innovations in a simple social media survey. The value of such an A/B test lies in the difference between its expected and its actual outcome: At Microsoft, only 1/3 of all changes tested in experiments turn out to be effective while 1/3 have a neutral and 1/3 even a negative impact.
If we could spark your interest and you are now looking for more information on the value of A/B tests for your organization, have a look at this Harvard Business Review article by Ron Kohavi, prior lead of experimentation at Airbnb and Microsoft, and Stefan Thomke, William Barclay Harding Professor of Business Administration at Harvard Business School (2017).